Published
- 4 min read
Alibaba QwQ-32B: Efficient AI Model and Reinforcement Learning
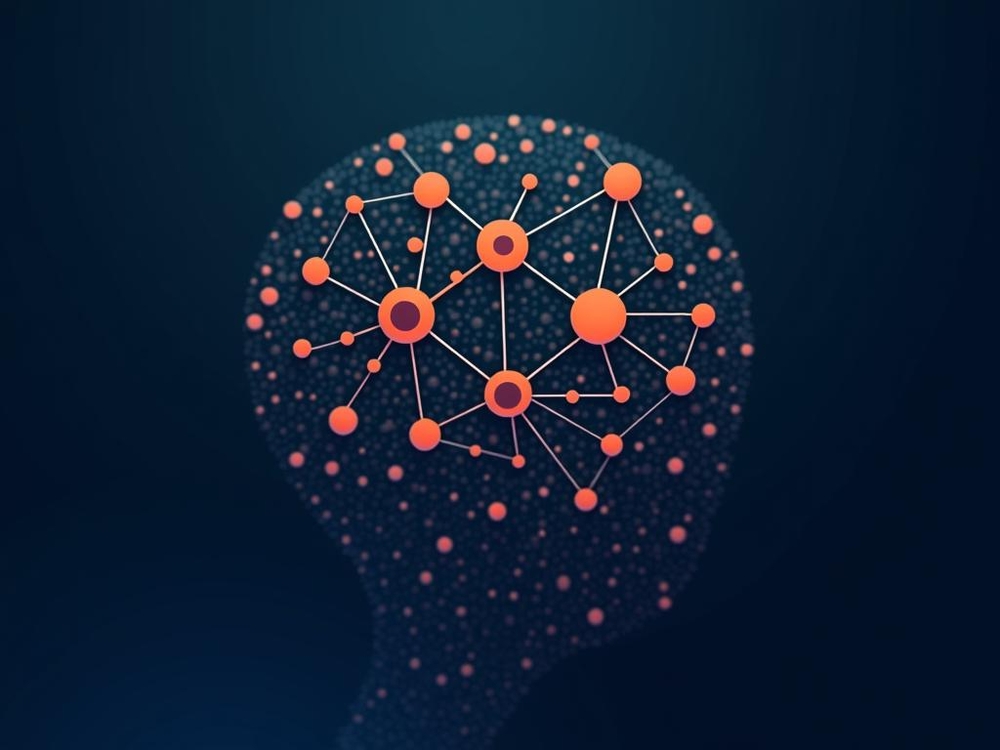
Alibaba’s new open source model QwQ-32B matches DeepSeek-R1 with way smaller compute requirements
My friends, today I want to talk about something truly remarkable in the world of artificial intelligence. When it comes to technological breakthroughs, we often think bigger is better. More parameters, more computing power, more resources. That’s been conventional wisdom.
Alibaba’s Qwen Team has just turned that wisdom on its head with their new QwQ-32B model. Make no mistake, this is an impressive achievement that deserves our attention and appreciation.
A smart answer to complex reasoning challenges
QwQ (which stands for Qwen-with-Questions) represents Alibaba’s thoughtful response to OpenAI’s original reasoning model. The name itself carries a certain playfulness, doesn’t it? “Questions with Questions.” The path to a good answer often begins with asking the right questions.
This 32-billion-parameter model can process up to 131,000 tokens at once. That’s about the length of a small novel. This capability helps in handling complex problems.
Doing more with less - the American way
I’ve always believed innovation isn’t just about raw power. It’s about efficiency and ingenuity. QwQ-32B embodies this principle beautifully.
While DeepSeek-R1 operates with a massive 671 billion parameters (activating 37 billion), QwQ-32B achieves comparable performance with dramatically fewer resources. This efficient engineering is like how the Wright brothers flew not by building the biggest engine but by understanding aerodynamics.
The multi-stage reinforcement learning approach is sophisticated:
- Focus first on math and coding fundamentals.
- Then enhance general capabilities.
All while keeping computational needs reasonable.
What this means for our future
For enterprise decision-makers exploring artificial intelligence options, QwQ-32B is an intriguing choice. The model delivers structured, context-aware insights without needing as much computing power as larger models.
The open-weight availability under Apache 2.0 license means organizations can tailor this technology for specific needs. This democratization of AI aligns with core innovation principles. The best ideas should be accessible and adaptable.
The reinforcement learning techniques used in QwQ-32B’s development show potential for more efficient paths to artificial general intelligence. Instead of just scaling up, Alibaba is exploring smarter and more nuanced methods.
Community response
The AI community is excited. New gurus praise QwQ-32B for its speed in inference and its performance relative to much larger models.
Access to comparable results with fewer resources is not just technical achievement. It might be a paradigm shift.
The road ahead
Qwen’s team sees QwQ-32B as just the beginning. Their roadmap includes:
- Further scaling reinforcement learning approaches.
- Integrating agents for long-term reasoning.
- Developing foundation models specifically for reinforcement learning.
- Moving toward sophisticated artificial general intelligence.
In closing, QwQ-32B is a reminder that progress doesn’t always require more. Sometimes, it requires smarter. The team at Alibaba shows that with strategic training and thoughtful engineering, we can achieve remarkable AI without extreme computational demands.
That’s a lesson we can all learn as we navigate this extraordinary technological moment together.
What is Alibaba QwQ-32B?
Alibaba’s QwQ-32B is a groundbreaking AI model developed by Alibaba’s Qwen Team. The QwQ-32B model, which stands for “Qwen-with-Questions,” consists of 32 billion parameters. It is designed to handle complex reasoning challenges by processing extensive data inputs of up to 131,000 tokens at once. QwQ-32B provides efficient AI model performance by doing more with fewer resources. This efficiency marks a significant shift from traditional AI approaches, which often prioritize larger, more computationally demanding models. The model is also noteworthy for its open-source availability, offering enterprises a flexible AI solution that can be customized for diverse applications.
How efficient is the QwQ-32B AI model?
The QwQ-32B model is highly efficient compared to other AI models. It achieves similar performance to larger models like DeepSeek-R1, which has 671 billion parameters, all while requiring significantly less computational power. This efficiency is achieved through a smart multi-stage reinforcement learning approach that focuses on core fundamentals like mathematics and coding. The strategy enhances the AI’s general capabilities while maintaining a manageable computational footprint. This allows QwQ-32B to deliver context-aware insights effectively, making it a viable option for enterprises that need powerful AI solutions without the extensive computational demands.
Summary
This article explored Alibaba’s revolutionary QwQ-32B AI model. It compared its efficiency to the larger DeepSeek-R1, discussed its multi-stage reinforcement learning approach, and highlighted how it could benefit enterprises seeking flexible AI solutions. The future holds further advancements as Qwen’s team plans to scale their methods and integrate more sophisticated AI technology. The reader can look forward to more innovations in AI models and approaches that balance power with efficiency.